Editor’s Note: The human learning process is extraordinarily complex. A multitude of factors influence this process positively or negatively. Dr. Ya-Wen Teng’s research in motivation and engagement is a welcome addition. She analyses factors that are significant predictors of success in online learning.
Students’ Backgrounds and Behaviors in a Web- Assisted Learning EnvironmentLoretta Ya-Wen Teng TaiwanAbstractThe study aimed to explore the predictors of the motivation to succeed, motivation for online participation, enjoyment in online learning and overall motivation to learn. The results indicated that gender and the enjoyment in online networking predicted the motivation for online participation and the motivation to succeed. The students from night school showed a better enjoyment in online networking while females and those who had noticed a difference of the course delivery format showed a higher overall motivation to learn. The results provided implications for planning and facilitating web-assisted learning to accommodate different students, especially at Taiwanese technical institutions. Keywords: Web-assisted learning, blended learning, learners’ backgrounds, online motivation, online enjoyment, online networking, web-assisted learning behaviors, blended learning behaviors, computer-assisted learning. IntroductionThe scope of research in online learning ranges from studying the instructors’ roles, students’ perceptions, satisfaction, performances to its effectiveness. In the literature, it is not uncommon to find discussions of how participants’ characteristics influence online learning. However, little attention has been drawn to how learners’ backgrounds may be inter-related to influence their behaviors in a web-assisted learning environment. In recommending the directions of research for online learning, Kennedy (2000) cautioned how student input variables might have confounded the results from existing studies. She suggested that the initial differences in online students needed to be considered to avoid misinterpreting any research findings when making inferences. It is thus important to investigate the learners’ backgrounds for a more comprehensive web-based instructional design, as well as understanding the complexity of how these factors may be inter-reliant to influence various learning behaviors in a web-assisted learning environment. Literature ReviewThe term “online learning” has been broadly adopted to describe courses or learning activities which are delivered fully online or featured by the integration of information technologies. Alternatively, it has also been described as “computer-assisted instruction,” “web-based learning,” or “internet-based learning.” The following review encompasses learning activities that were conducted purely online or in a hybrid mode mixed with online and face-to-face instructions. Gender and Age with Online Learning In the literature of online learning, gender and age are among the most widely discussed in identifying perceived or measured learning. The results have been mixed in various studies. Shaw and Peter (2000) concluded that there were no significant differences in how older and younger students reacted to asynchronous networked learning. Similarly, Lu, Yu, and Liu (2003) found that there were no age and gender differences in how the students performed in a web-based course. However, a difference in how non-traditional and traditional college students learned in an online environment was reported by Miller and Lu (2003) from surveying a group of online instructors. While Wojciechowski and Palmer (2005) found that older students performed better in online classes, in studying the level of engagement of online students, Garland and Martin (2003) discovered that male students accessed the course website more often while their female counterparts participated in more online group activities. In another study to investigate how age, gender, ability and motivation related to the achievement in an online course, older students were found to be more motivated in web-based learning, but gender did not significantly predict performance (Hoskins & van Hooff, 2005). Despite the lack of a significant difference, males in this study demonstrated more knowledge in using web discussions but were less comfortable in web dialoguing. Family Backgrounds and Online Learning Tinto (1993) stated that student attributes and family background affected the initial level of commitment to goal attainment. Riehl (1994) concurred that those with one or more college-educated parents were more likely to stay in college. From investigating the factors affecting the development of life-long learning in Malaysia, Alwi, Syed, Mohamad, and Said (2005) concluded that when parents valued education more, their children put more efforts in learning. Research showed that first-generation college students experienced college life differently from their traditional-aged peers (Terenzini, Springer, Yaeger, Pascarella & Nora, 1996). Aside from being predisposed to a lower academic achievement (Riehl, 1994), first generation students were likely to receive less support from families for education (York-Anderson & Bowman, 1991). On the contrary, Pratt and Skaggs (1989) did not support the notion that first-generation college students were at greater risk. A finding from their study led to a conclusion that this student population had a stronger institutional commitment and did not differ in goal attainment as well as in academic and social integration. With online learning, Williams and Hellman (2004) found that first-generation college students reported a lower level of self-regulation for online learning than their second-generation counterparts. A study on distance learning in Malaysia also showed that family background and attributes affected distance learning (Dzakira, & Idrus, 2003). As for computer skills, Martinez and Mead (1988) found that there was a positive correlation between parents’ educational background and students’ competency in using computers to learn. In measuring the acquisition of information, Buzzetto-More and Sweat-Guy (2006) discovered a marginal correlation between parents’ level of education and freshmen’s technological readiness in a Historically Black University. Finally, Barker and Wendel (2001) found that strong parental support contributed positively to the success in online learning. The Social Aspect of Online Learning The development of online communities was found to contribute positively to the quality and quantity of learning (Picciano, 2002). This notion was supported by numerous studies. For instance, Levin, Waddoups, Levin, and Buell (2001) reported that an environment of high student-to-student interactions contributed effectively to online learning. The positive aspect of creating a sense of community was also proposed by Wiesenberg and Hutton (1996), who attested that online networking increased the students’ ability to think independently. Lam, Cheng, and McNaught (2005) empirically proved that active online participation induced positive learning outcomes. They also highly valued online communication as beneficial to the learning process. In Brook and Oliver’s (2003) review of students’ characteristics that led to the development of an online community, they summarized that socialization tended to be gender-based. This position was confirmed by one of Richardson and Swan’s (2003) research finding that gender accounted for online students’ perception of social presence. These researchers found a high correlation between the students’ perception of social presence and perceived learning. In other words, more learning occurred when a sense of bonding were perceived among the learners. As Gunawardena and Zittle (1997) indicated, social presence in online settings was related to learner satisfaction. On the contrary, lacking such an experience can create a barrier in online learning (Muilenburg & Berge, 2005). Motivation and Enjoyment in Online Learning As Muilenburg and Berge (2005) pointed out, the absence of motivation from learners prevented success in online learning. A group of online graduate students shared their insight that motivation impacted the online learning experiences (Song, Singleton, Hill & Koh, 2003). A similar observation was reported in Bullen’s (2007) study which noted a positive influence of intrinsic motivation on active participation in online tasks. Kennedy (2000) considered motivation the key to successful online learning and encouraged future researchers to study how it could relate to online learning experience and outcomes. Interestingly, Prince and Stern (2002) found that older students who participated voluntarily were more likely to put in more efforts in online learning. In another instance, Hoskins and van Hooff (2005) witnessed older students’ inclination to post online and male students’ preference in online communication. The enjoyment for online participation appeared to be another vital factor for effective online learning. A study on the acceptance of internet-based learning medium highlighted perceived enjoyment as a predictor of the intrinsic motivation for internet-based learning (Lee, Cheung, & Chen, 2005). This proposition was not a novelty because Salmon (1998) had previously advocated for online moderators to foster enjoyment. According to Pintrich and De Groot (1990), those who perceived learning as interesting tended to be more cognitively involved in the process. More evidences were presented by Wu and Hiltz (2004), who concluded that the perception of learning was strongly related to online learners’ level of enjoyment. Similarly, Muilenburg and Berge (2005) found a connection between enjoyment in online learning and social interactions. Obviously, learners’ backgrounds can influence their experience with online or web-assisted learning. While the backgrounds can each impact learner’s experience and perception with online learning, the question of how and which of these variables can combine to influence online learners’ experience remains unanswered. Purpose of the StudyIn this study, the author sought to examine how gender, age, type of high school attended, and parents’ educational background of college students influenced how they behaved in web-assisted learning environments. Specifically, the study was designed to answer the following questions: In a web-assisted learning environment, what predicted the motivation to succeed in this course, motivation for online participation, and the enjoyment in online learning from a combination of variables? what predicted the students’ overall motivation to learn from a combination of variables? The operational definitions of motivation and enjoyment in online learning are described in the “method” section.
MethodParticipants One hundred and eighty eight Taiwanese technical college students who enrolled in “Physiology and Mental Health,” a general education requirement taught by the researcher were surveyed in the study. The agreement to be surveyed was obtained and the survey was administered at the last class session. Among them, more than half (61.7) participated in the online component of the course. There were more female students (77.7%) and most of them were freshmen (65.2%). More of them were from the day classes (58.5%) and most of them went to vocational high schools (79.3%). Those whose parents were in the labor (38.3%) and business (31.9%) industries represented most of the sample. In addition, 89.4% of them were first- generation college students whose parents did not attend college. There were 81.3% of them who agreed that they had to contribute to family income. A number of them reported working for more than 25 hours per week (62.9%). Those who worked over 35 hours a week counted for 41.1% of the sample. Instrumentation The measurement for the study was a survey of 30 questions made up of a demographic component yielding background information and Likert-scaled questions for assessing various learning behaviors. All questions were conceptualized first for their relevance to the measured variables before being delivered to a panel of five experts for a test of validity. These experts were college instructors of social sciences and were familiar with web-based learning. Through this process, five seemingly repetitive questions were dropped and changes in wording were made in accordance with the recommendations of the experts. The correlations among all of the variables represented by the survey questions were performed and those with high correlations (r >.50) were grouped together to avoid collinearity in subsequent analyses. Doing so resulted in the formation of the three variables listed below: The motivation to succeed in class The motivation for online participation The enjoyment in online networking
The motivation to succeed was composed of “the desire to receive a good grade for the course” and “the desire to earn extra credits by participating in online discussions.” The motivation for online participation was a composite scale of “time spent in completing online assignments,” “logging in weekly to complete online assignments,” “feeling that participating in online discussions improved the understanding of course content and key points,” “enjoying online discussions,” and “acquiring different viewpoints from group discussions.” The summated scale of “enjoyment in online networking” was computed from “feeling that participating in online discussions improved the understanding of course content” “feeling that online course content and activities were helpful” “online discussions helped me connect with classmates,” and “online discussions helped me connect with the instructor.” Finally, the variables measuring the students’ overall motivation to learn were formed from combining “motivation to succeed in class,” and “motivation for online participation.” The internal consistency of each of the composite variables was obtained with a Cronbach’s α of .69 for motivation to succeed in class, α = .84 for motivation to participate in online activities, α = .87 for enjoyment in online networking, and finally α = .87 for the overall motivation to learn. Although the internal consistency of the variable “motivation to succeed in class” fell below the general acceptable level of .70, it was kept as a valid summated scale for its high conceptual agreement among the items, and proximity to the acceptable level suggested by content experts. Procedure In the beginning of the semester, the students were informed of the “blended” or “mixed” instructional/learning format of the course. Lecture presentations, both in Microsoft PowerPoint and video formats were made available through the university’s online course management system, Wisdom Master (http://demo.learn.com.tw/1000410147/product2.htm), developed by a local technology corporation in Taiwan. The access to the course was password protected and the verifications for course registrations were required by the system, and performed by the instructor. An orientation of the online participation was provided during the first four weeks of the semester and reinforced as needed thereafter. After the completion of the online course registration process, the students could access the course web site http://elearning.ctust.edu.tw/ to participate in class activities. The students were encouraged to participate in online forums with topics related to the course content. Each discussion was initiated by the instructor and announced in the announcement area of the course system prior to its being made available. Each of the six discussions lasted for about two weeks. The students were guided for their online participation in terms of the quality and quantity of the posts. To increase the interactions among the students, they were asked to respond to others in addition to posting their own thoughts. In order to foster the development of a learning community, an introductory forum with warm-up exercises and a social forum were offered for the students to get acquainted with their cross sectional peers. Online office hours were offered regularly throughout the semester using the instant chat function of the courseware. External links to a free photo sharing web site and personality tests were provided for course related activities. The photo site was created to help personalize the learning experience. With the online personality tests, the students were asked to post their reflections in the discussion area before depositing the test results online in the course system’s assignment folder. The evaluation of the students’ online activities was recorded and reviewed in class during mid-term and again, close to the end of the semester. The online participation was counted as a part of the students’ assignment grade, which contributed 30% to their course performance. ResultsMultiple regressions were performed to identify the predictors for the students’ motivation to succeed in the course, the motivation to participate in online activities, the enjoyment in online networking and their overall motivation to learn. Motivation to Succeed in the Course The analysis showed that 30% of the variance in the motivation to succeed was predicted from the eight variables combined. These variables were the enjoyment in online networking, parents’ educational background, family background, the type of high school attended, gender, hours of work each week, age, and whether or not they were from the day or evening school. The effect size was large. The variable of “parents’ educational background” referred to whether or not one or both parents had received college education. The question on family background asked the students to identify if they were from single-parent households. As a result, gender, beta = .166, p = .018, and enjoyment in online networking, beta = .498, p <.001, were found to significantly predict the motivation to succeed in the course (R = .58; adjusted R² = .30). This result is presented in Table 1. 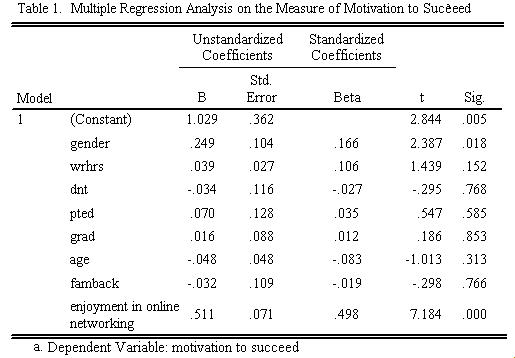
Motivation for Online Participation Gender (Beta = .150, p = .007) and the enjoyment in online networking (Beta = .673, p < .001) combined to significantly predict the motivation for online participation (R = .769; adjusted R² = .573) when these two variables were entered into a regression model simultaneously, along with the following six other variables: hours of work for each week, taking day or evening classes, parents’ educational background, the type of high school attended, age, and family background. About 57% of the variance in the motivation for online participation was predicted from a combination of these variables, which was a very large effect size. This result is summarized in Table 2. 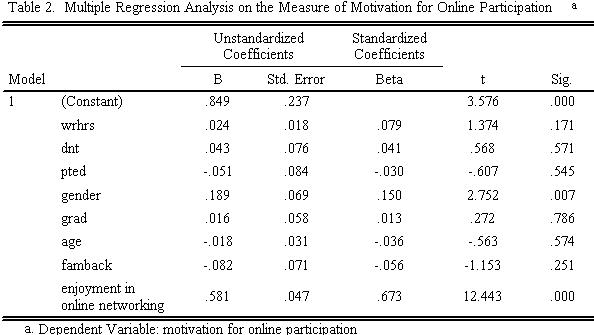 The Enjoyment in Online Networking To identify the predictors for the enjoyment in online networking, seven variables were entered in the regression equation. These variables included gender, hours of work for each week, attending the day or evening school, parents’ educational background, the type of high school attended, family background, and perceiving a difference in the course delivery method. The result indicated that attending the day or evening schools, beta = .283, p = .001, significantly predicted the enjoyment in online networking (R = .442, adjusted R² = .163). Variance predicted from these variables combined was 16%, which was a medium-large effect size. Table 3 shows this result. 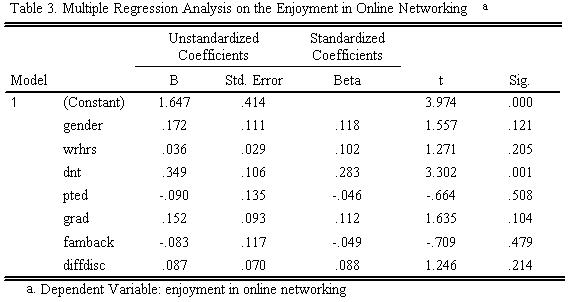
The Overall Motivation to Learn The following 10 variables were considered in a regression model to determine the predictors for the overall motivation to learn: gender, hours of work for each week, attending the day or evening school, parents’ educational background, the type of high school attended, age, family background, parents’ occupation, whether they chose to attend this institution voluntarily, and perceiving the difference in the course delivery method. The analysis showed that 23% of the variance was predicted from these variables combined, which was a medium-large effect size. Furthermore, the combination of gender (Beta = .202, p = .006) and perceiving the difference in the course delivery method (Beta = .203, p = .003) significantly predicted the motivation to learn (R = .524, adjusted R² = .232). Table 4 shows this result. DiscussionGender emerged as an important predictor for the students’ overall motivation to learn, participate and succeed in online activities. In general, female participants showed higher motivation in all three investigated areas. It was possible that female students were more attracted to learn in a non-traditional web-assisted course, when online dialoguing was an activity of their preference. In fact, research findings have revealed that females were better in building the affective and social aspects of an online learning community (Im & Lee, 2003). These findings indicated that female students who enjoyed networking in the online environment were more driven to participate and do well in online learning activities. The results also suggested that the female participants’ enjoyment in online involvement led to their more satisfying experience in developing relationships with their peers and instructor. 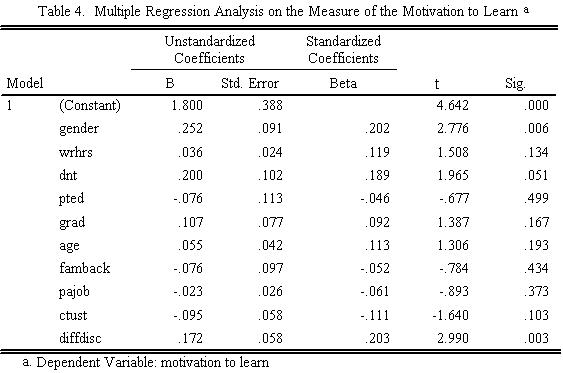
Interestingly, females who had observed the unique course delivery method had a higher overall motivation to learn. Apparently, these students paid more attention to the course requirement. Besides participating in online forums, the students were required to collaborate in class for structured group discussions by rotating to assume different roles. Qu, Wang and Johnson (2005) associated motivation with the learners’ focus of attention. Zhang, Cheng, He and Huang (2003) also predicted motivation from attentive behaviors. In a motivational model presented by Keller (1987), getting learners’ attention was the key to improve their motivational level. Similarly, Tremblay and Gardner (1995) related attention, along with other factors, to motivation. It was possible that the female students’ enjoyment in online participation contributed positively to their being more mindful with the collaborative feature of this course. This in turn could have influenced their motivation for optimal performance because according to Reeve (1989), enjoyment contributed to the persistence in an activity. The fact that many evening students at Taiwanese technical institutions work and go to school full-time makes it difficult for them to interact with their classmates and instructors. In the Taiwanese higher educational system, only full-time attendance is allowed. Thus, the online forums might have become an alternative platform for socializing and networking under this circumstance. This may also explain why the evening students enjoyed online networking more compared to the students from the day classes. One explanation was that these students had developed mutual support among themselves for their online participation. Research has highlighted evening college students’ need for learning support (Yan, 2006). Unfortunately, research also showed that many of the evening students expressed a lower level of satisfaction with the advising they had received from faculty (Dunker & Belcastro, 2003). It is important to note that in this study, the participants from the evening classes were all freshmen; therefore, the online networking experience might have extended an opportunity outside of the limited class time for those who were new to college to connect with peers, instructors and the new college life. Apparently, the participants’ family background, referred to as growing up with both parents or in single-parent households, did not influence their enjoyment in online participation and motivation of various contexts. Students from single-parent households were not pre-disposed to a less motivated or enjoyable web-assisted learning experience. Interestingly, parents’ educational background did not influence students’ learning behaviors when considered among other factors. The motivation and enjoyment in online networking of first-generation college students were not undermined by their parents’ having not received college education. The study contributes to the research of blended learning which features the mixture of traditional and online instruction. According to the results, planning for online activities that are enjoyable is the key to achieve motivated learning. Additionally, web-assisted instruction can be a viable option for the development of social or learning networks outside of the regular class meetings. Future research can be designed to identify male students’ leaning styles as associated with web-assisted learning, for promoting their level of motivation and enjoyment. Specifically, exploring the strategies for involving male learners in online communication can be helpful in planning web-enhanced instruction. ReferencesAlwi, M. R., Syed, S. B., Moamad, S. H., & Said, J. (2005, November). Factors facilitating and hindering the development of Malaysian life long learning commitment. Paper presented at the ICDE International Conference, New Delhi, India. Barker, K., & Wendel, T. (2001). E-learning: studying Canada’s virtual secondary schools (Research Series #8). Kwlowna, B.C.: Society for the Advancement of Excellence in Education. Bullen, M. (2007). Participation and critical thinking in online university distance education. Journal of Distance Education, 13(2). Buzzetoo-More, N., & Sweat-Guy, R. (2006, October). Technology backgrounds of freshmen students at historical black universities. Paper presented at the Global Digital Business Association Meeting, Las Vegas, NV. Brook, C., & Oliver, R. (2003). Online learning communities: investigating a design framework. Australian Journal of Educational Technology, 19(2), 139-160. Dunker, R. E., & Belcastro, F. P. (2003). A comparison of daytime and evening students’ satisfaction with their faculty academic advising program. Community College Journal of Research and Practice, 17(2), 123-130. Dzakira, H. & Idrus, R. M. (2003). Teacher-learner interactions in distance education: a case of two Malaysian universities. Turkish Online Journal of Distance Education, 4(3). Garland, D., & Martin, B. (2003). Does gender affect online learning? Women in Higher Education, 8(1). Gunawardena, C. L., & Zittle, F. J. (1997). Social presence as a predictor of satisfaction with a computer-mediated conferencing environment. American Journal of Distance Education, 11(3), 8-26. Hoskins, S. L., & van Hooff, J. C. (2005). Motivation and ability: which students use online leaning and what influence does it have on their achievement? British Journal of Educational Technology, 36(2), 177-192. Im, Y., & Lee, O. (2003). Pedagogical implications of online discussions for preservice teacher training. Journal of Research of Technology in Education, 36(2), 155-170. Keller, J. M. (1987). Development and use of the ARCs model of motivational design. Journal of Instructional Development, 10(3), 2-10. Kennedy, C. (2000). What influences student learning in an online course. (ERIC Document Reproduction Service No. ED 466 238) Lam, P., Cheng, K., & McNaught, C. (2005). Asynchronous online discussion: empirical evidence on quantity and quality. In P. Kommers & G. Richards (Eds.), Proceedings of the World Conference on Educational Multimedia, Hypermedia and Telecommunications (EDMEDIA) (pp. 3209-3215). Chesapeake, VA: AACE. Lee, M. K. O., Cheung, C. M. K., & Chen, Z. (2005). Acceptance of internet-based learning medium: the role of extrinsic and intrinsic motivation. Information & Management, 42, 1095-1104. Levin, S. R., Waddoups, G. L., Levin, J, & Buell, J. (2001). Highly interactive and effective online learning environments for teacher professional development. International Journal of Educational Technology, 2(2), 2001. Lu, J., Yu, C. S., & Liu, C. (2003). Learning style, learning patterns, and learning performance in a WebCT-based course. Information & Management, 40(6), 497-507. Martinez, M. E., & Mead, N. A. (1988). Computer competence: The first national assessment (NAEP Report 17-CC-01). Princeton, NJ: Educational Testing Service. Miller, M., & Lu, M. Y. (2003). Serving non-traditional students in e-learning environments: building successful communities in the virtual campus. Educational Media International, 40(182), 163-169. Muilenburg, L. Y., & Berge, Z. L. (2005). Student barriers to online learning: a factor analytic study. Distance Education, 26(1), 29-48. Picciano, A. G. (2002). Beyond student perceptions: issues of interactions, presence, and performance in an online course. JALN, 6(1). Pintrich, P. R., & De Groot, E. V. (1990). Motivation and self-regulated learning components of classroom academic performance. Journal of Educational Psychology, 82(1), 33-40. Pratt, P. A., & Skaggs, C. T. (1989). First-generation college students: are they at greater risk for attrition than their peers? Research in Rural Education, 6(2), 31-34. Prince, D., & Stern, P. (2002). Online learning students: characteristics, satisfaction, and implications for future planning. A summary of findings from the system development project. Research Report. Washington State Board for Community and Technical Colleges. (ERIC Document Reproduction Services No. ED 481 385). Qu, L., Wang, N., Johnson, W. L. (2005). Detecting the learner’s motivational states in an interactive learning environment. In C. K. Looi et al. (Eds.). Artificial Intelligence in Education (pp. 309-330). Amsterdam: IOS Press. Reeve, J. (1989). The interest-enjoyment distinction in intrinsic motivation. Motivation and Emotion, 13(2). Richardson, J. C., & Swan, K. (2003). Examining social presence in online courses in relation to students’ perceived learning and satisfaction. JALN, 7(1). Riehl, R. J. (1994), The academic preparation, aspirations, and first-year performance of first-generation students. College and University, 70(1), 14-19. Salmon, G. (1998). Developing learning through effective online moderation. Active Learning, December, 3-9. Shaw, G. P., & Peter, W. (2000). The use of asynchronous learning networks in nutrition education: student attitude, experiences, and performances. JALN, 4(1). Song, L., Singleton, E. S., Hill, J. R., & Koh, M, H. (2003). Improving online learning: student perceptions of useful and challenging characteristics. The Internet and Higher Education, 7(1), 59-70. Tinto, V. (1993). Leaving college: rethinking causes and cures of student attrition (2nd ed.). Chicago: University of Chicago Press. Tremblay, P. F., & Gardner, R. C. (1995). Expanding the motivation construct in language learning. The Modern Language Journal, 79(4), 505-518. Terenzini, P. T., Springer, L., Yaeger, P. M. Pascarella, E. T., & Nora, A. (1996). First-generation college students: characteristics, experiences, and cognitive development. Research in Higher Education, 37(1), 1-22. Wiesenberg, F., & Hutton, S. (1996). Teaching a graduate program using computer-mediated conferencing software. Journal of Distance Education, 11(1). 83-100. Williams, D. E., & Hellman, C. M. (2004). Differences in self-regulation for online learning between first- and second-generation college students. Research in Higher Education, 45(1), 71-82. Wojciechowski, A., & Palmer, L. B. (2005). Individual student characteristics: can any be predictors of success in online classes? Online Journal of Distance Learning Administration, III(II). Wu, D., & Hiltz, S. R. (2004). Predicting learning from asynchronous online discussions. JALN, 8(2), 139-152. Yan, C. H. (2006). Factors affecting the state anxiety level of higher education students in Macau: the impact of trait anxiety and self-esteem. Assessment and Evaluation in Higher Education, 31(6), 709-725. York-Anderson, D. C., & Bowman, S. L. (1991). Assessing the college knowledge of first-generation and second-generation college students. Journal of College Student Development, 32(2), 116-122. Zhang, G., Cheng, Z., He, A., & Huang, T. A. (2003, September). WWW-based learning motivation detecting system. Paper presented at the International Workshop on “Research Directions and Challenges Problems in Advanced Information System Engineering, Akita, Japan. About the AuthorDr. Loretta Ya-Wen Teng is a faculty member of behavioral and social sciences at Central Taiwan University of Science and Technology. She has led and participated in many web-assisted intercultural projects and interdisciplinary initiatives. With a background in counseling, some of her current research projects include the study of counseling supervisory effectiveness, online cross-cultural collaboration, and interactive instruction. More information on Dr. Teng can be obtained from her website http://kms.ctust.edu.tw/teng/LT/. e-mail: lorettateng@gmail.com
|